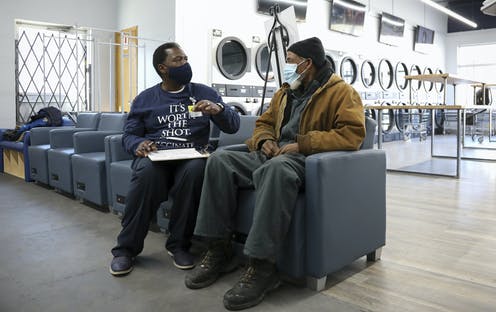
Public health officials are focusing on the 30% of the eligible population that remains unvaccinated against COVID-19 as of the end of October 2021, and that requires figuring out where those people are and why they are unvaccinated.
People remain unvaccinated for many reasons, including belief in unfounded conspiracy theories about the disease, the vaccines or both; distrust of the medical establishment; concerns about risks and side effects; fear of needles; and difficulty accessing vaccines. To target their messaging and outreach geographically and according to the type of hesitancy, public health officials need good data to guide their efforts. Traditional survey methods are helpful but tend to be expensive.
Another approach is to assess vaccine hesitancy through the lens of social media. As an artificial intelligence researcher, I analyze social media data using machine learning. My latest research, conducted with graduate student Sara Melotte and accepted for publication in the journal PLOS Digital Health, predicts the degree of vaccine hesitancy at the ZIP code level in U.S. metropolitan areas by analyzing geo-located tweets.
We found that by processing geo-located Twitter data using readily available machine learning techniques, we could more accurately predict vaccine hesitancy by ZIP code than by using attributes of ZIP codes like average home price and number of health care and social services facilities.
Table of Contents
The limits of surveys
Surveys, such as a Gallup COVID-19 survey launched in 2020, estimate vaccine hesitancy levels in the general population by polling a representative sample with a Yes/No vaccine hesitancy question: If a Food and Drug Administration-approved vaccine to prevent coronavirus/COVID-19 was available right now at no cost, would you agree to be vaccinated? The estimated vaccine hesitancy is the percentage of individuals who respond “No.” As demonstrated both in our research and work by others, factors such as location, income and education levels all correlate with vaccine hesitancy.
A general disadvantage of such surveys is that detailed questions are expensive to administer. Sample sizes tend to be small due to cost constraints and non-response rates. The latter has been exacerbated recently by political polarization. Computational social science methods, which use computer algorithms to analyze large amounts of data, are another option, but they can have trouble interpreting noisy social media text to glean insights.
Mining Twitter
Our work takes on the challenge of using publicly available Twitter data to accurately predict vaccine hesitancy in a given ZIP code. We focused on ZIP codes in major metropolitan areas, which are known for high tweeting activity. Users also enable GPS more often in these areas.
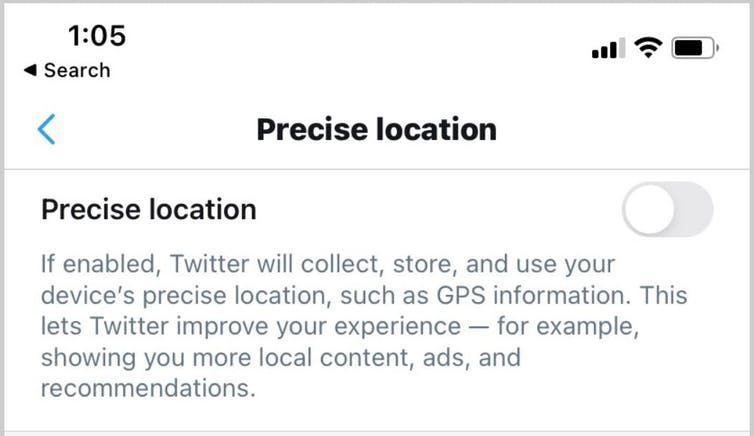
Screenshot by The Conversation U.S., CC BY-ND
As a first step, we downloaded all the tweets from a publicly available dataset called GeoCoV19, which filters tweets to be as relevant to COVID-19 as possible. Next, using peer-reviewed methodology, we filtered the tweets down to GPS-enabled tweets from the top metropolitan areas. We then randomly split the tweets into a training set and a test set. The former was used to develop the model, while the latter was used to evaluate the model.
Training a model to predict the vaccine hesitancy of a ZIP code is like drawing a straight line through a set of points so that the line comes as close as possible to the center of the points, known as a line of best fit. The line indicates the trend in the data. The first step is converting the raw text of tweets into data points.
[The Conversation’s science, health and technology editors pick their favorite stories. Weekly on Wednesdays.]
Recently developed deep neural networks are able to automatically convert the text into data points so that tweets with similar meanings are closer together. We essentially used such a network to convert our tweets to data points and then trained our machine learning model on those data points. We validated our model using the Gallup COVID-19 survey results.
Our method performed better at predicting high levels of vaccine hesitancy than methods that only use generic features, like average home prices within the ZIP code, rather than social media data. We also showed our model to be effective in the presence of tweets that aren’t related to vaccines or COVID-19. The GeoCov19 dataset is good but includes many tweets that are not relevant specifically to vaccines and a small – but non-trivial – fraction that are not relevant to COVID-19 at all.
Early detection and prevention
In research currently undergoing peer review, we developed algorithms that automatically mine potential causes of vaccine hesitancy, and their extent, from social media. Our preliminary analysis confirms that while some causes are the result of conspiracy theories and misinformation, others are informed by legitimate concerns such as potential vaccine side effects.
We expect that people with these concerns may be much more amenable to getting vaccinated if they are presented with reliable sources of information that assuage their fears. In the future, public health officials could use machine learning for early detection of vaccine hesitancy on social media. Then they could use algorithms to automatically distribute targeted information and go on the offense against the spread of health-related misinformation.
Such future digital public health systems could lead to healthier outcomes, both in the physical and digital realms.
Mayank Kejriwal does not work for, consult, own shares in or receive funding from any company or organization that would benefit from this article, and has disclosed no relevant affiliations beyond their academic appointment.